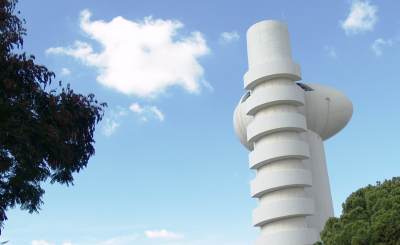
UChicago: David Awschalom, Hannes Bernien, Liang Jiang, & Tian Zhong, Pritzker School of Molecular Engineering
WIS: Rotem Arnon-Friedman, Nir Davidson, Ofer Firstenberg, Serge Rosenblum, Ephraim Shahmoon
Networks of classical computers have revolutionized the world in the past few decades. Future quantum networks will likewise enable important new applications, such as secure quantum communication, distributed quantum sensing, and delegated quantum computation. An outstanding challenge in building a global quantum network is overcoming the loss and noise in the optical communication channels used to transfer fragile quantum states. To overcome this challenge, we will build quantum repeaters consisting of two key ingredients – entanglement creation between quantum memories to suppress loss errors and heralded entanglement distillation to suppress noise and operation errors.
Through this project, the PIs aim to demonstrate efficient entanglement generation between photons and memory nodes and entanglement swapping to create entanglement between distant memory nodes. In addition, they will theoretically optimize entanglement distillation protocols and experimentally demonstrate proof-of-concept entanglement distillation to correct realistic noises. Finally, these efforts will culminate in the integration of entanglement creation and entanglement distillation protocols at the UChicago-Argonne test-bed, which spans quantum nodes separated by more than 100 km
WIS: Tali Dekel, Sarel Fleishman
TTIC: Jinbo Xu
Proteins are responsible for all the molecular processes essential for life on Earth. Optimization and design of synthetic proteins with desired functions is a long-standing challenge that has been studied for many years and has tremendous implications in real-world applications including drug design, gene editing and DNA sequencing. Fleishman (Weizmann) has developed the state-of-the-art atomistic methods PROSS and FuncLib for optimizing protein stability and activity, but the reliable design of large changes in activity or completely new ones is an unaddressed challenge. Xu (TTIC) developed the first deep-learning (DL) method for protein structure prediction, revolutionizing the field and inspiring DeepMind’s AlphaFold. Dekel (Weizmann) developed cutting-edge DL methods for image and video analysis. Together, the PIs will develop and test DL methods for optimizing enzymes and binding proteins and for the design of completely new ones. The methods will be applied to enzymes that are required for sustainability and energy applications and to potentially therapeutic antibodies. Successful completion of the project will furnish novel, experimentally validated DL approaches for protein design and broaden participation among students from under-represented groups in both Chicago and Israel.
UChicago: Matthew Tirrell, Pritzker School of Molecular Engineering
WIS: Yonina Eldar, Jacob Klein
The properties of macromolecular surface phases such as polyelectrolyte brushes or lipid bilayers play a crucial role in many applications, particularly in biolubrication. The Tirrell (Chicago) and Klein (Weizmann) groups have studied, respectively, the boundary lubrication properties of polyelectrolyte brushes and of lipid bilayers using surface force balance (SFB) techniques. These provide unique details at the sub-nanometer spatial and interaction scales, and have shed strong light on how parameters including concentration and valency of surrounding salt, pH, areal density of the polyelectrolyte brushes, and the composition, phase state and hydration level of the lipid bilayers, modulate lubrication.
SFB experiments, however, while providing exquisitely-sensitive information on the lubrication, are challenging and time-consuming, which precludes them from examining all but a small fraction of the relevant parameter space. The PIs will exploit machine learning approaches, in which Eldar (Weizmann) is an expert, to reveal the combinations of parameters which will enable us to optimize such lubrication processes. They will use large data sets, covering a large parameter space, generated by much simpler experiments, such as atomic force microscopy (AFM) imaging and force measurements for the polyelectrolyte brushes, and by molecular dynamics simulations for the lipid bilayers, to train machine learning algorithms using methods including random forests, gradient boosting and deep neural networks. Following training, the algorithm performance will be validated on additional data; after running many combinations of parameters they will test the optimal machine-learning-predicted lubrication parameters by full-scale SFB experiments on both polyelectrolyte brushes and lipid bilayers.
UChicago: Cong Ma & Jingshu Wang, Department of Statistics
WIS: Amos Tanay
UChicago: Dmitri Talapin, Department of Chemistry
WIS: Sivan Refaely Abramson, Dan Oron
UChicago: Matthew Stephens, Departments of Statistics and Human Genetics
WIS: Eran Segal